Home page of NSF model¶
By Xin Wang, Shinji Takaki, Junichi Yamagishi
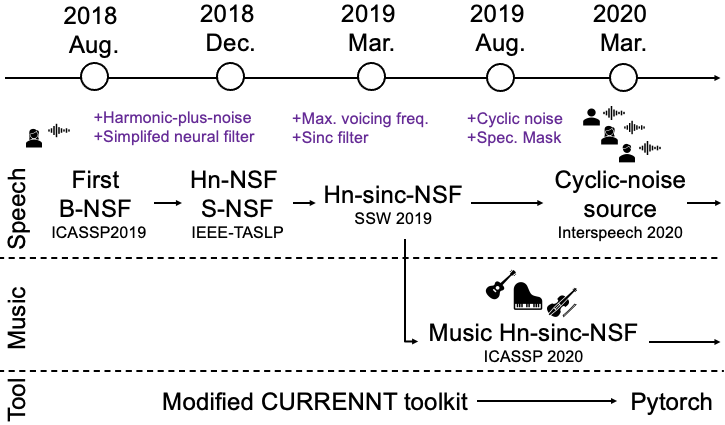
This the home site of neural source-filter (NSF) waveform models.
This site hosts the sample pages of all the NSF models illustrated in the figure. The latest work comes first. If you have any questions or suggestions, please send email to wangxin ~a~t~ nii ~dot~ ac ~dot~ jp.
- Links:
Sample pages of other projects: github page
Home page of Xin Wang on github
Pytorch project¶
Here is the Pytorch re-implementation of NSF models:
Notes:
This repository incldues the cyclic-noise-NSF, hn-sinc-NSF, hn-NSF;
Demo script and pre-trained models on CMU_ARCTIC database are included;
Tutorials (Jupyter notebooks) are available in this tutorial sub-directory;
Our NSF papers used the old CURRENNT implementation, not this Pytorch one (see code and scripts)
Comments and suggestions are welcome!
Cyclic-noise-NSF¶
Using cyclic noise as source signals for NSF-based speech waveform modeling
Audio sample pages:
Samples on CMU_ARCTIC database -> Cyclic-noise-NSF (CMU samples)
Samples on VCTK database -> Cyclic-noise-NSF (VCTK samples)
Paper link:
Wang, X. & Yamagishi, J. Using Cyclic Noise as the Source Signal for Neural Source-Filter-Based Speech Waveform Model. in Proc. Interspeech 1992–1996. doi:10.21437/Interspeech.2020-1018
BibTex:
@inproceedings{wang2020cyclic,
address = {ISCA},
author = {Wang, Xin and Yamagishi, Junichi},
booktitle = {Proc. Interspeech},
doi = {10.21437/Interspeech.2020-1018},
pages = {1992--1996},
publisher = {ISCA},
title = {{Using Cyclic Noise as the Source Signal for Neural Source-Filter-Based Speech Waveform Model}},
url = {http://www.isca-speech.org/archive/Interspeech{\_}2020/abstracts/1018.html},
year = {2020}
}
Hn-sinc-NSF for music¶
Transferring neural speech waveform synthesizers to musical instrument sounds generation
This work applies NSF models to music instrumental audios generation. A single model can be trained to generate different types of instruments: brass, string, and woodwind instruments.
Audio sample page:
Audio samples on URMP database -> Hn-sinc-NSF music
Paper:
Zhao, Y., Wang, X., Juvela, L. & Yamagishi, J. Transferring neural speech waveform synthesizers to musical instrument sounds generation. in Proc. ICASSP 6269–6273 (IEEE, 2020). doi:10.1109/ICASSP40776.2020.9053047
BibTex:
@inproceedings{Zhao2020,
author = {Zhao, Yi and Wang, Xin and Juvela, Lauri and Yamagishi, Junichi},
booktitle = {Proc. ICASSP},
doi = {10.1109/ICASSP40776.2020.9053047},
pages = {6269--6273},
title = {{Transferring neural speech waveform synthesizers to musical instrument sounds generation}},
url = {https://ieeexplore.ieee.org/document/9053047/},
year = {2020}
}
Hn-sinc-NSF¶
Neural Harmonic-plus-Noise Waveform Model with Trainable Maximum Voice Frequency for Text-to-Speech Synthesis
This new model enhances hn-NSF with trainable maximum voiced frequency (MVF) and sinc-based high/low-pass FIR filters
Audio sample page:
Audio samples on ATR Ximera F009 voice -> Hn-sinc-NSF
Paper:
Wang, X. & Yamagishi, J. Neural Harmonic-plus-Noise Waveform Model with Trainable Maximum Voice Frequency for Text-to-Speech Synthesis. in Proc. SSW 1–6 (ISCA, 2019). doi:10.21437/SSW.2019-1
BibTex:
@inproceedings{Wang2019,
address = {ISCA},
author = {Wang, Xin and Yamagishi, Junichi},
booktitle = {Proc. SSW},
doi = {10.21437/SSW.2019-1},
pages = {1--6},
publisher = {ISCA},
title = {{Neural Harmonic-plus-Noise Waveform Model with Trainable Maximum Voice Frequency for Text-to-Speech Synthesis}},
url = {http://www.isca-speech.org/archive/SSW{\_}2019/abstracts/SSW10{\_}O{\_}1-1.html},
year = {2019}
}
Hn-NSF and s-NSF¶
Neural Source-Filter Waveform Models for Statistical Parametric Speech Synthesis
This work introduces two new NSF models: s-NSF with simplified neural filter blocks and hn-NSF with harmonic-plus-noise structure. It also explains the details of NSF, which cannot fit into the ICASSP paper.
Audio sample page:
Audio sample on ATR Ximera F009 voice -> Hn-NSF and s-NSF
Paper:
Wang, X., Takaki, S. & Yamagishi, J. Neural Source-Filter Waveform Models for Statistical Parametric Speech Synthesis. IEEE/ACM Trans. Audio, Speech, Lang. Process. 28, 402–415 (2020), DOI:10.1109/TASLP.2019.2956145
BibTex:
@article{wangNSFall,
author = {Wang, Xin and Takaki, Shinji and Yamagishi, Junichi},
doi = {10.1109/TASLP.2019.2956145},
journal = {IEEE/ACM Transactions on Audio, Speech, and Language Processing},
pages = {402--415},
title = {{Neural Source-Filter Waveform Models for Statistical Parametric Speech Synthesis}},
url = {https://ieeexplore.ieee.org/document/8915761/},
volume = {28},
year = {2020}
}
Baseline NSF¶
Neural source-filter-based waveform model for statistical parametric speech synthesis
This is frst NSF model, a by-product of unsuccessful reproduction of Parallel WaveNet. Hence, the model uses similar dilated CNN blocks as WaveNet.
Audio sample page:
Audio sample on ATR Ximera F009 and CMU_ARCTIC SLT -> First NSF
Paper:
Wang, X., Takaki, S. & Yamagishi, J. Neural source-filter-based waveform model for statistical parametric speech synthesis. in Proc. ICASSP 5916–5920 (2019). DOI:10.1109/ICASSP.2019.8682298
BibTex:
@inproceedings{wang2018neural,
author = {Wang, Xin and Takaki, Shinji and Yamagishi, Junichi},
booktitle = {Proc. ICASSP},
doi = {10.1109/ICASSP.2019.8682298},
pages = {5916--5920},
publisher = {IEEE},
title = {{Neural Source-filter-based Waveform Model for Statistical Parametric Speech Synthesis}},
url = {https://ieeexplore.ieee.org/document/8682298/},
year = {2019}
}
Acknowledgement¶
This project cannot be done without the help of reviewers, readers, colleagues, and all the friends:
The original CURRENNT toolkit is the work of Felix Weninger and colleagues, a fantastic toolkit using CUDA/THRUST. It is available on Sourceforge;
WORLD vocoder is the work of Dr. Morise;
CMU_ARCTIC database is provided by Language Technologies Institute, Carnegie Mellon University;
URMP database is provided by AIR lab, University of Rochester;
This work can improved. Your feedback is welcome!
Note¶
Audio other than the natural samples on this website are distributed with under a Creative Commons Attribution Non-Commercial NoDerivatives 4.0 (CC BY-NC-ND 4.0) license.
